Unnormalized Log Probability - RNNIs There any RNN method used for Object detectionHow is the LSTM RNN forget gate calculated?How to train the same RNN over multiple series?Find most important inputs of LSTM-RNN for multivariate time series modelingLoss function for an RNN used for binary classificationWhy the RNN has input shape error?Input and output Dimension of LSTM RNNWhat is the advantage of using RNN with fixed timestep length over Neural Network?Need to make an multivariate RNN, confused about input shape?RNN for prediciting Development over time
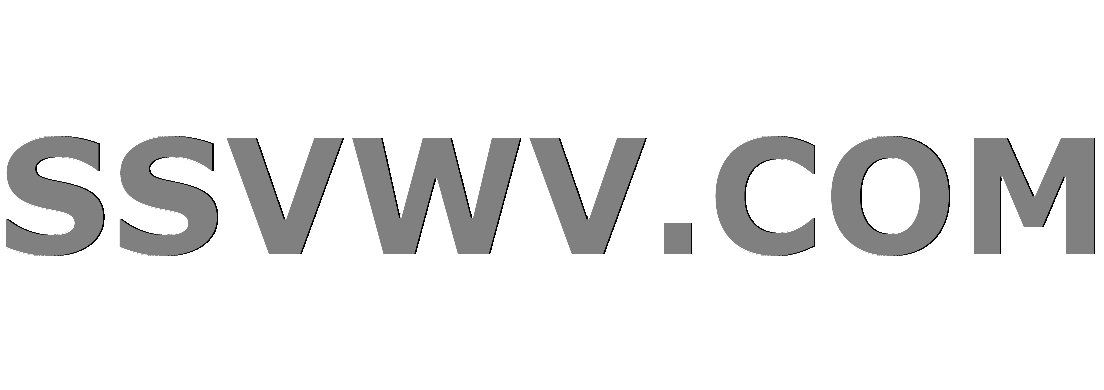
Multi tool use
Is there any evidence that Cleopatra and Caesarion considered fleeting to India to escape the Romans?
Quoting Keynes in a lecture
Giving feedback to someone without sounding prejudiced
Why does a 97 / 92 key piano exist by Bösendorfer?
Should I assume I have passed probation?
Integral Notations in Quantum Mechanics
Can I say "fingers" when referring to toes?
What fields between the rationals and the reals allow a good notion of 2D distance?
How to preserve electronics (computers, iPads and phones) for hundreds of years
No results when searching for contacts on facet value
On definitions and explicit examples of pure-injective modules
Does Doodling or Improvising on the Piano Have Any Benefits?
How many people need to be born every 8 years to sustain population?
The Digit Triangles
Given this phrasing in the lease, when should I pay my rent?
Remove specific words in a string
Is it feasible to let a newcomer play the "Gandalf"-like figure I created for my campaign?
Assign a specific color to vector layer based on RGB codes in attribute table
What is the Difference Between Formulating the Answer via Quadratic Formula and Factoring?
If Captain Marvel (MCU) were to have a child with a human male, would the child be human or Kree?
Would a primitive species be able to learn English from reading books alone?
Logistic function with a slope but no asymptotes?
Is there a reason to prefer HFS+ over APFS for disk images in High Sierra and/or Mojave?
Why is participating in the European Parliamentary elections used as a threat?
Unnormalized Log Probability - RNN
Is There any RNN method used for Object detectionHow is the LSTM RNN forget gate calculated?How to train the same RNN over multiple series?Find most important inputs of LSTM-RNN for multivariate time series modelingLoss function for an RNN used for binary classificationWhy the RNN has input shape error?Input and output Dimension of LSTM RNNWhat is the advantage of using RNN with fixed timestep length over Neural Network?Need to make an multivariate RNN, confused about input shape?RNN for prediciting Development over time
$begingroup$
I am going through the deep learning book by Goodfellow. In the RNN section I am stuck with the following:
RNN is defined like following:
And the equations are :
Now the $O^(t)$ above is considered as unnormalized log probability. But if this is true, then the value of $O^(t)$ must be negative because,
Probability is always defined as a number between 0 and 1, i.e, $Pin[0,1]$, where brackets denote closed interval. And $log(P) le 0$ on this interval. But in the equations above, nowhere this condition that $O^(t) le 0$ is explicitly enforced.
What am I missing!
deep-learning lstm recurrent-neural-net
$endgroup$
add a comment |
$begingroup$
I am going through the deep learning book by Goodfellow. In the RNN section I am stuck with the following:
RNN is defined like following:
And the equations are :
Now the $O^(t)$ above is considered as unnormalized log probability. But if this is true, then the value of $O^(t)$ must be negative because,
Probability is always defined as a number between 0 and 1, i.e, $Pin[0,1]$, where brackets denote closed interval. And $log(P) le 0$ on this interval. But in the equations above, nowhere this condition that $O^(t) le 0$ is explicitly enforced.
What am I missing!
deep-learning lstm recurrent-neural-net
$endgroup$
add a comment |
$begingroup$
I am going through the deep learning book by Goodfellow. In the RNN section I am stuck with the following:
RNN is defined like following:
And the equations are :
Now the $O^(t)$ above is considered as unnormalized log probability. But if this is true, then the value of $O^(t)$ must be negative because,
Probability is always defined as a number between 0 and 1, i.e, $Pin[0,1]$, where brackets denote closed interval. And $log(P) le 0$ on this interval. But in the equations above, nowhere this condition that $O^(t) le 0$ is explicitly enforced.
What am I missing!
deep-learning lstm recurrent-neural-net
$endgroup$
I am going through the deep learning book by Goodfellow. In the RNN section I am stuck with the following:
RNN is defined like following:
And the equations are :
Now the $O^(t)$ above is considered as unnormalized log probability. But if this is true, then the value of $O^(t)$ must be negative because,
Probability is always defined as a number between 0 and 1, i.e, $Pin[0,1]$, where brackets denote closed interval. And $log(P) le 0$ on this interval. But in the equations above, nowhere this condition that $O^(t) le 0$ is explicitly enforced.
What am I missing!
deep-learning lstm recurrent-neural-net
deep-learning lstm recurrent-neural-net
edited Mar 17 at 9:38


Siong Thye Goh
1,367519
1,367519
asked Mar 17 at 8:40
user3001408user3001408
360146
360146
add a comment |
add a comment |
2 Answers
2
active
oldest
votes
$begingroup$
You are right in a sense that it is better to be called log of unnormalized probability. This way, the quantity could be positive or negative. For example, $textlog(0.5) < 0$ and $textlog(12) > 0$ are both valid log of unnormalized probabilities. Here, in more detail:
Probability: $P(i) = e^o_i/sum_k=1^Ke^o_k$ (using softmax as mentioned in Figure 10.3 caption, and assuming $mathbfo=(o_1,..,o_K)$ is the output of layer before softmax),
Unnormalized probability: $tildeP(i) = e^o_i$, which can be larger than 1,
Log of unnormalized probability: $textlogtildeP(i) = o_i$, which can be positive or negative.
$endgroup$
add a comment |
$begingroup$
You are right, nothing stop $o_k^(t)$ from being nonnegative, the keyword here is "unnormalized".
If we let $o_k^(t)=ln q_k^(t)$
$$haty^(t)_k= fracexp(o^(t)_k)sum_k=1^K exp(o^(t)_k) = fracq_k^(t)sum_k=1^K q_k^(t)$$
Here $q_k^(t)$ can be any positive number, they will be normalized to be sum to $1$.
$endgroup$
add a comment |
Your Answer
StackExchange.ifUsing("editor", function ()
return StackExchange.using("mathjaxEditing", function ()
StackExchange.MarkdownEditor.creationCallbacks.add(function (editor, postfix)
StackExchange.mathjaxEditing.prepareWmdForMathJax(editor, postfix, [["$", "$"], ["\\(","\\)"]]);
);
);
, "mathjax-editing");
StackExchange.ready(function()
var channelOptions =
tags: "".split(" "),
id: "557"
;
initTagRenderer("".split(" "), "".split(" "), channelOptions);
StackExchange.using("externalEditor", function()
// Have to fire editor after snippets, if snippets enabled
if (StackExchange.settings.snippets.snippetsEnabled)
StackExchange.using("snippets", function()
createEditor();
);
else
createEditor();
);
function createEditor()
StackExchange.prepareEditor(
heartbeatType: 'answer',
autoActivateHeartbeat: false,
convertImagesToLinks: false,
noModals: true,
showLowRepImageUploadWarning: true,
reputationToPostImages: null,
bindNavPrevention: true,
postfix: "",
imageUploader:
brandingHtml: "Powered by u003ca class="icon-imgur-white" href="https://imgur.com/"u003eu003c/au003e",
contentPolicyHtml: "User contributions licensed under u003ca href="https://creativecommons.org/licenses/by-sa/3.0/"u003ecc by-sa 3.0 with attribution requiredu003c/au003e u003ca href="https://stackoverflow.com/legal/content-policy"u003e(content policy)u003c/au003e",
allowUrls: true
,
onDemand: true,
discardSelector: ".discard-answer"
,immediatelyShowMarkdownHelp:true
);
);
Sign up or log in
StackExchange.ready(function ()
StackExchange.helpers.onClickDraftSave('#login-link');
);
Sign up using Google
Sign up using Facebook
Sign up using Email and Password
Post as a guest
Required, but never shown
StackExchange.ready(
function ()
StackExchange.openid.initPostLogin('.new-post-login', 'https%3a%2f%2fdatascience.stackexchange.com%2fquestions%2f47460%2funnormalized-log-probability-rnn%23new-answer', 'question_page');
);
Post as a guest
Required, but never shown
2 Answers
2
active
oldest
votes
2 Answers
2
active
oldest
votes
active
oldest
votes
active
oldest
votes
$begingroup$
You are right in a sense that it is better to be called log of unnormalized probability. This way, the quantity could be positive or negative. For example, $textlog(0.5) < 0$ and $textlog(12) > 0$ are both valid log of unnormalized probabilities. Here, in more detail:
Probability: $P(i) = e^o_i/sum_k=1^Ke^o_k$ (using softmax as mentioned in Figure 10.3 caption, and assuming $mathbfo=(o_1,..,o_K)$ is the output of layer before softmax),
Unnormalized probability: $tildeP(i) = e^o_i$, which can be larger than 1,
Log of unnormalized probability: $textlogtildeP(i) = o_i$, which can be positive or negative.
$endgroup$
add a comment |
$begingroup$
You are right in a sense that it is better to be called log of unnormalized probability. This way, the quantity could be positive or negative. For example, $textlog(0.5) < 0$ and $textlog(12) > 0$ are both valid log of unnormalized probabilities. Here, in more detail:
Probability: $P(i) = e^o_i/sum_k=1^Ke^o_k$ (using softmax as mentioned in Figure 10.3 caption, and assuming $mathbfo=(o_1,..,o_K)$ is the output of layer before softmax),
Unnormalized probability: $tildeP(i) = e^o_i$, which can be larger than 1,
Log of unnormalized probability: $textlogtildeP(i) = o_i$, which can be positive or negative.
$endgroup$
add a comment |
$begingroup$
You are right in a sense that it is better to be called log of unnormalized probability. This way, the quantity could be positive or negative. For example, $textlog(0.5) < 0$ and $textlog(12) > 0$ are both valid log of unnormalized probabilities. Here, in more detail:
Probability: $P(i) = e^o_i/sum_k=1^Ke^o_k$ (using softmax as mentioned in Figure 10.3 caption, and assuming $mathbfo=(o_1,..,o_K)$ is the output of layer before softmax),
Unnormalized probability: $tildeP(i) = e^o_i$, which can be larger than 1,
Log of unnormalized probability: $textlogtildeP(i) = o_i$, which can be positive or negative.
$endgroup$
You are right in a sense that it is better to be called log of unnormalized probability. This way, the quantity could be positive or negative. For example, $textlog(0.5) < 0$ and $textlog(12) > 0$ are both valid log of unnormalized probabilities. Here, in more detail:
Probability: $P(i) = e^o_i/sum_k=1^Ke^o_k$ (using softmax as mentioned in Figure 10.3 caption, and assuming $mathbfo=(o_1,..,o_K)$ is the output of layer before softmax),
Unnormalized probability: $tildeP(i) = e^o_i$, which can be larger than 1,
Log of unnormalized probability: $textlogtildeP(i) = o_i$, which can be positive or negative.
edited Mar 17 at 13:29
answered Mar 17 at 9:36
EsmailianEsmailian
1,601114
1,601114
add a comment |
add a comment |
$begingroup$
You are right, nothing stop $o_k^(t)$ from being nonnegative, the keyword here is "unnormalized".
If we let $o_k^(t)=ln q_k^(t)$
$$haty^(t)_k= fracexp(o^(t)_k)sum_k=1^K exp(o^(t)_k) = fracq_k^(t)sum_k=1^K q_k^(t)$$
Here $q_k^(t)$ can be any positive number, they will be normalized to be sum to $1$.
$endgroup$
add a comment |
$begingroup$
You are right, nothing stop $o_k^(t)$ from being nonnegative, the keyword here is "unnormalized".
If we let $o_k^(t)=ln q_k^(t)$
$$haty^(t)_k= fracexp(o^(t)_k)sum_k=1^K exp(o^(t)_k) = fracq_k^(t)sum_k=1^K q_k^(t)$$
Here $q_k^(t)$ can be any positive number, they will be normalized to be sum to $1$.
$endgroup$
add a comment |
$begingroup$
You are right, nothing stop $o_k^(t)$ from being nonnegative, the keyword here is "unnormalized".
If we let $o_k^(t)=ln q_k^(t)$
$$haty^(t)_k= fracexp(o^(t)_k)sum_k=1^K exp(o^(t)_k) = fracq_k^(t)sum_k=1^K q_k^(t)$$
Here $q_k^(t)$ can be any positive number, they will be normalized to be sum to $1$.
$endgroup$
You are right, nothing stop $o_k^(t)$ from being nonnegative, the keyword here is "unnormalized".
If we let $o_k^(t)=ln q_k^(t)$
$$haty^(t)_k= fracexp(o^(t)_k)sum_k=1^K exp(o^(t)_k) = fracq_k^(t)sum_k=1^K q_k^(t)$$
Here $q_k^(t)$ can be any positive number, they will be normalized to be sum to $1$.
answered Mar 17 at 9:37


Siong Thye GohSiong Thye Goh
1,367519
1,367519
add a comment |
add a comment |
Thanks for contributing an answer to Data Science Stack Exchange!
- Please be sure to answer the question. Provide details and share your research!
But avoid …
- Asking for help, clarification, or responding to other answers.
- Making statements based on opinion; back them up with references or personal experience.
Use MathJax to format equations. MathJax reference.
To learn more, see our tips on writing great answers.
Sign up or log in
StackExchange.ready(function ()
StackExchange.helpers.onClickDraftSave('#login-link');
);
Sign up using Google
Sign up using Facebook
Sign up using Email and Password
Post as a guest
Required, but never shown
StackExchange.ready(
function ()
StackExchange.openid.initPostLogin('.new-post-login', 'https%3a%2f%2fdatascience.stackexchange.com%2fquestions%2f47460%2funnormalized-log-probability-rnn%23new-answer', 'question_page');
);
Post as a guest
Required, but never shown
Sign up or log in
StackExchange.ready(function ()
StackExchange.helpers.onClickDraftSave('#login-link');
);
Sign up using Google
Sign up using Facebook
Sign up using Email and Password
Post as a guest
Required, but never shown
Sign up or log in
StackExchange.ready(function ()
StackExchange.helpers.onClickDraftSave('#login-link');
);
Sign up using Google
Sign up using Facebook
Sign up using Email and Password
Post as a guest
Required, but never shown
Sign up or log in
StackExchange.ready(function ()
StackExchange.helpers.onClickDraftSave('#login-link');
);
Sign up using Google
Sign up using Facebook
Sign up using Email and Password
Sign up using Google
Sign up using Facebook
Sign up using Email and Password
Post as a guest
Required, but never shown
Required, but never shown
Required, but never shown
Required, but never shown
Required, but never shown
Required, but never shown
Required, but never shown
Required, but never shown
Required, but never shown
SyXNI 2oDaIUZdsY41ipCnDI9MT,rleK8c3qXiEoqElgN2 hT evrRFpaDkFx N5C,C