Determining multivariate least squares with constraintNonlinear least squaresImplementation of Partial Least Squares (PLS)?Ordinary Least Squares (OLS) with RLinknon linear Least Squares with model function given by ODE'sComputing least squares errorLeast squares approximationLeast squares fit to find unknown coefficientsWeighted orthogonal least squares without constant termLinear least squaresNonlinear Weighted Total Least Squares
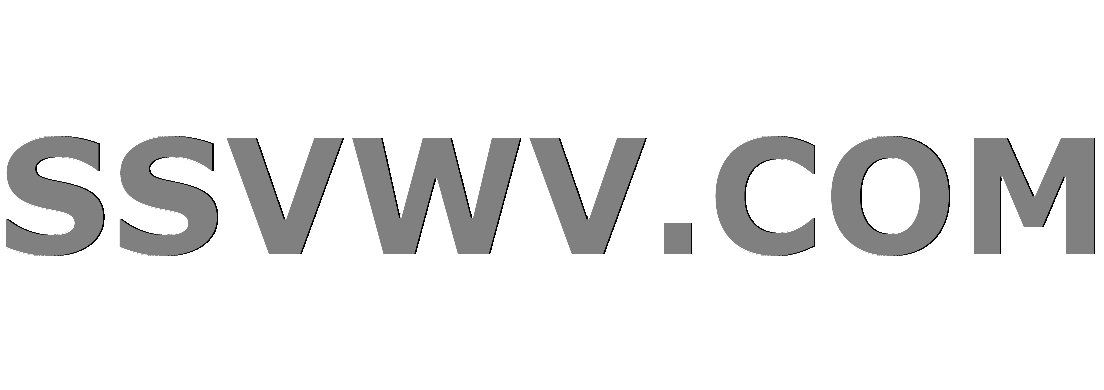
Multi tool use
Mysql fixing root password
Pressure to defend the relevance of one's area of mathematics
Toggle Overlays shortcut?
Modify locally tikzset
How does a Swashbuckler rogue "fight with two weapons while safely darting away"?
Why do Ichisongas hate elephants and hippos?
Can solid acids and bases have pH values? If not, how are they classified as acids or bases?
Binary Numbers Magic Trick
Upright [...] in italics quotation
Counterexample: a pair of linearly ordered sets that are isomorphic to subsets of the other, but not isomorphic between them
Why is the origin of “threshold” uncertain?
Do I have to worry about players making “bad” choices on level up?
Phrase for the opposite of "foolproof"
Options leqno, reqno for documentclass or exist another option?
How can Republicans who favour free markets, consistently express anger when they don't like the outcome of that choice?
How to stop co-workers from teasing me because I know Russian?
Do generators produce a fixed load?
Illegal assignment from SObject to Contact
Are Boeing 737-800’s grounded?
Unexpected email from Yorkshire Bank
What was the "glowing package" Pym was expecting?
Can not tell colimits from limits
Was there a Viking Exchange as well as a Columbian one?
Python "triplet" dictionary?
Determining multivariate least squares with constraint
Nonlinear least squaresImplementation of Partial Least Squares (PLS)?Ordinary Least Squares (OLS) with RLinknon linear Least Squares with model function given by ODE'sComputing least squares errorLeast squares approximationLeast squares fit to find unknown coefficientsWeighted orthogonal least squares without constant termLinear least squaresNonlinear Weighted Total Least Squares
$begingroup$
I have some composition data for a geological sample and its constituent minerals. I want to estimate the proportions of the minerals that make up the bulk sample. This is essentially a mass balance problem. Each variable is pseudo-independent (but constrained by constant sum).
I'm starting with the matrix form $a.x=b$,
where, $a$ is the array $elemental~composition~vector times mineral ~species$, $x$ is the column vector of mineral proportions, and $b$ is the bulk composition. The key constraint with this kind of problem is that
$0 leq x_j leq 1~~~~~(j=1,...m)$
and
$sum_j=1^m x_j=1$
here is some example data
a=63.1545, 64.3049, 100., 37.1417, 32.4026, 30.0382, 30.7033, 0., 36.5444, 0., 0., 0., 0.0034,
0.0016, 0.01, 0., 0.6641, 2.35946, 26.91, 0., 0., 0.297125, 0., 0., 0., 51.3026,
17.8683, 21.096, 0., 11.2387, 14.3019, 6.46115, 0.34805, 0., 14.368, 0., 0., 0., 0.,
0.0223, 0.1191, 0., 30.6859, 32.0779, 1.5471, 1.5014, 0., 11.5299, 0., 0., 0., 43.3621,
0., 0., 0., 0.94408, 0.6131, 0.0986, 0., 0., 0., 0., 0., 0., 2.1039,
0., 0., 0., 1.14598, 1.82486, 0.03695, 0.00935, 0., 0.2312, 0., 0., 0., 0.0166,
0.625667, 9.77073, 0., 1.35584, 0.0558, 0.05635, 0., 0., 0.079275, 0., 0., 0., 0.018,
0., 2.80603, 0., 10.7388, 0.0245429, 27.7454, 2.19443, 55.08, 10.5478, 56.03, 78.13, 10.44, 0.0262,
15.7365, 0.0874667, 0., 1.89652, 8.54851, 0.00475, 0., 0., 0.09665, 0., 0., 0., 0.0115,
0., 0., 0., 0.16636, 0., 0., 0.0293, 42.4, 0.0114, 0., 0., 0., 0.0617
b=65.67, 0.52, 14.77, 5.418, 0.13, 0.3, 3.22, 2.05, 6.07, 0.13
If I use the LeastSquares
function I get
lsq = LeastSquares[a, b]
0.331681, 0.283916, 0.166439, 0.172393, 0.0586919,
0.0795275,
0.00802007, 0.00244529, -0.030593, -0.0157832, -0.0220087,
-0.00294087, -0.0363984
This result looks OK, except negative proportions are not allowed and the sum does not equal 1.
Total@lsq
0.99539
How do I implement linear least squares fitting for multivariate data under the constraint that values lie between 0 to 1 and sum to 1 in Mathematica?
Also how can I derive the residuals for the fit?
fitting
$endgroup$
|
show 2 more comments
$begingroup$
I have some composition data for a geological sample and its constituent minerals. I want to estimate the proportions of the minerals that make up the bulk sample. This is essentially a mass balance problem. Each variable is pseudo-independent (but constrained by constant sum).
I'm starting with the matrix form $a.x=b$,
where, $a$ is the array $elemental~composition~vector times mineral ~species$, $x$ is the column vector of mineral proportions, and $b$ is the bulk composition. The key constraint with this kind of problem is that
$0 leq x_j leq 1~~~~~(j=1,...m)$
and
$sum_j=1^m x_j=1$
here is some example data
a=63.1545, 64.3049, 100., 37.1417, 32.4026, 30.0382, 30.7033, 0., 36.5444, 0., 0., 0., 0.0034,
0.0016, 0.01, 0., 0.6641, 2.35946, 26.91, 0., 0., 0.297125, 0., 0., 0., 51.3026,
17.8683, 21.096, 0., 11.2387, 14.3019, 6.46115, 0.34805, 0., 14.368, 0., 0., 0., 0.,
0.0223, 0.1191, 0., 30.6859, 32.0779, 1.5471, 1.5014, 0., 11.5299, 0., 0., 0., 43.3621,
0., 0., 0., 0.94408, 0.6131, 0.0986, 0., 0., 0., 0., 0., 0., 2.1039,
0., 0., 0., 1.14598, 1.82486, 0.03695, 0.00935, 0., 0.2312, 0., 0., 0., 0.0166,
0.625667, 9.77073, 0., 1.35584, 0.0558, 0.05635, 0., 0., 0.079275, 0., 0., 0., 0.018,
0., 2.80603, 0., 10.7388, 0.0245429, 27.7454, 2.19443, 55.08, 10.5478, 56.03, 78.13, 10.44, 0.0262,
15.7365, 0.0874667, 0., 1.89652, 8.54851, 0.00475, 0., 0., 0.09665, 0., 0., 0., 0.0115,
0., 0., 0., 0.16636, 0., 0., 0.0293, 42.4, 0.0114, 0., 0., 0., 0.0617
b=65.67, 0.52, 14.77, 5.418, 0.13, 0.3, 3.22, 2.05, 6.07, 0.13
If I use the LeastSquares
function I get
lsq = LeastSquares[a, b]
0.331681, 0.283916, 0.166439, 0.172393, 0.0586919,
0.0795275,
0.00802007, 0.00244529, -0.030593, -0.0157832, -0.0220087,
-0.00294087, -0.0363984
This result looks OK, except negative proportions are not allowed and the sum does not equal 1.
Total@lsq
0.99539
How do I implement linear least squares fitting for multivariate data under the constraint that values lie between 0 to 1 and sum to 1 in Mathematica?
Also how can I derive the residuals for the fit?
fitting
$endgroup$
2
$begingroup$
My usual soapbox speech: Why not consult a statistician first and then figure out how to implement an appropriate process in Mathematica? Consider stats.stackexchange.com/questions/267014/….
$endgroup$
– JimB
Mar 20 at 14:53
$begingroup$
@JimB precisely because someone will tell me that I can't use continuum methods with compositional data. The constant sum problem is considered a vague oddity in geological circles and largely ignored (for better or worse).
$endgroup$
– geordie
Mar 20 at 15:26
$begingroup$
I'm sure there must be more to it than "They won't let me do what I want!" Compositional data analysis has a pretty standard set of statistical approaches. Chemists use it all the time. These approaches are many times called "mixture designs": itl.nist.gov/div898/handbook/pri/section5/pri54.htm. Maybe you've been talking to the wrong folks.
$endgroup$
– JimB
Mar 20 at 15:49
$begingroup$
Like I said, geologists don't. Perhaps it has to do with the general 'noisiness' of geoscience datasets. Most other sciences are able to rely on fairly precise numbers.
$endgroup$
– geordie
Mar 20 at 16:22
1
$begingroup$
"Most other sciences are able to rely on fairly precise numbers." Ha, ha. You're funny!
$endgroup$
– JimB
Mar 20 at 16:30
|
show 2 more comments
$begingroup$
I have some composition data for a geological sample and its constituent minerals. I want to estimate the proportions of the minerals that make up the bulk sample. This is essentially a mass balance problem. Each variable is pseudo-independent (but constrained by constant sum).
I'm starting with the matrix form $a.x=b$,
where, $a$ is the array $elemental~composition~vector times mineral ~species$, $x$ is the column vector of mineral proportions, and $b$ is the bulk composition. The key constraint with this kind of problem is that
$0 leq x_j leq 1~~~~~(j=1,...m)$
and
$sum_j=1^m x_j=1$
here is some example data
a=63.1545, 64.3049, 100., 37.1417, 32.4026, 30.0382, 30.7033, 0., 36.5444, 0., 0., 0., 0.0034,
0.0016, 0.01, 0., 0.6641, 2.35946, 26.91, 0., 0., 0.297125, 0., 0., 0., 51.3026,
17.8683, 21.096, 0., 11.2387, 14.3019, 6.46115, 0.34805, 0., 14.368, 0., 0., 0., 0.,
0.0223, 0.1191, 0., 30.6859, 32.0779, 1.5471, 1.5014, 0., 11.5299, 0., 0., 0., 43.3621,
0., 0., 0., 0.94408, 0.6131, 0.0986, 0., 0., 0., 0., 0., 0., 2.1039,
0., 0., 0., 1.14598, 1.82486, 0.03695, 0.00935, 0., 0.2312, 0., 0., 0., 0.0166,
0.625667, 9.77073, 0., 1.35584, 0.0558, 0.05635, 0., 0., 0.079275, 0., 0., 0., 0.018,
0., 2.80603, 0., 10.7388, 0.0245429, 27.7454, 2.19443, 55.08, 10.5478, 56.03, 78.13, 10.44, 0.0262,
15.7365, 0.0874667, 0., 1.89652, 8.54851, 0.00475, 0., 0., 0.09665, 0., 0., 0., 0.0115,
0., 0., 0., 0.16636, 0., 0., 0.0293, 42.4, 0.0114, 0., 0., 0., 0.0617
b=65.67, 0.52, 14.77, 5.418, 0.13, 0.3, 3.22, 2.05, 6.07, 0.13
If I use the LeastSquares
function I get
lsq = LeastSquares[a, b]
0.331681, 0.283916, 0.166439, 0.172393, 0.0586919,
0.0795275,
0.00802007, 0.00244529, -0.030593, -0.0157832, -0.0220087,
-0.00294087, -0.0363984
This result looks OK, except negative proportions are not allowed and the sum does not equal 1.
Total@lsq
0.99539
How do I implement linear least squares fitting for multivariate data under the constraint that values lie between 0 to 1 and sum to 1 in Mathematica?
Also how can I derive the residuals for the fit?
fitting
$endgroup$
I have some composition data for a geological sample and its constituent minerals. I want to estimate the proportions of the minerals that make up the bulk sample. This is essentially a mass balance problem. Each variable is pseudo-independent (but constrained by constant sum).
I'm starting with the matrix form $a.x=b$,
where, $a$ is the array $elemental~composition~vector times mineral ~species$, $x$ is the column vector of mineral proportions, and $b$ is the bulk composition. The key constraint with this kind of problem is that
$0 leq x_j leq 1~~~~~(j=1,...m)$
and
$sum_j=1^m x_j=1$
here is some example data
a=63.1545, 64.3049, 100., 37.1417, 32.4026, 30.0382, 30.7033, 0., 36.5444, 0., 0., 0., 0.0034,
0.0016, 0.01, 0., 0.6641, 2.35946, 26.91, 0., 0., 0.297125, 0., 0., 0., 51.3026,
17.8683, 21.096, 0., 11.2387, 14.3019, 6.46115, 0.34805, 0., 14.368, 0., 0., 0., 0.,
0.0223, 0.1191, 0., 30.6859, 32.0779, 1.5471, 1.5014, 0., 11.5299, 0., 0., 0., 43.3621,
0., 0., 0., 0.94408, 0.6131, 0.0986, 0., 0., 0., 0., 0., 0., 2.1039,
0., 0., 0., 1.14598, 1.82486, 0.03695, 0.00935, 0., 0.2312, 0., 0., 0., 0.0166,
0.625667, 9.77073, 0., 1.35584, 0.0558, 0.05635, 0., 0., 0.079275, 0., 0., 0., 0.018,
0., 2.80603, 0., 10.7388, 0.0245429, 27.7454, 2.19443, 55.08, 10.5478, 56.03, 78.13, 10.44, 0.0262,
15.7365, 0.0874667, 0., 1.89652, 8.54851, 0.00475, 0., 0., 0.09665, 0., 0., 0., 0.0115,
0., 0., 0., 0.16636, 0., 0., 0.0293, 42.4, 0.0114, 0., 0., 0., 0.0617
b=65.67, 0.52, 14.77, 5.418, 0.13, 0.3, 3.22, 2.05, 6.07, 0.13
If I use the LeastSquares
function I get
lsq = LeastSquares[a, b]
0.331681, 0.283916, 0.166439, 0.172393, 0.0586919,
0.0795275,
0.00802007, 0.00244529, -0.030593, -0.0157832, -0.0220087,
-0.00294087, -0.0363984
This result looks OK, except negative proportions are not allowed and the sum does not equal 1.
Total@lsq
0.99539
How do I implement linear least squares fitting for multivariate data under the constraint that values lie between 0 to 1 and sum to 1 in Mathematica?
Also how can I derive the residuals for the fit?
fitting
fitting
edited Mar 20 at 16:12
user64494
3,65311222
3,65311222
asked Mar 20 at 13:14


geordiegeordie
2,0491630
2,0491630
2
$begingroup$
My usual soapbox speech: Why not consult a statistician first and then figure out how to implement an appropriate process in Mathematica? Consider stats.stackexchange.com/questions/267014/….
$endgroup$
– JimB
Mar 20 at 14:53
$begingroup$
@JimB precisely because someone will tell me that I can't use continuum methods with compositional data. The constant sum problem is considered a vague oddity in geological circles and largely ignored (for better or worse).
$endgroup$
– geordie
Mar 20 at 15:26
$begingroup$
I'm sure there must be more to it than "They won't let me do what I want!" Compositional data analysis has a pretty standard set of statistical approaches. Chemists use it all the time. These approaches are many times called "mixture designs": itl.nist.gov/div898/handbook/pri/section5/pri54.htm. Maybe you've been talking to the wrong folks.
$endgroup$
– JimB
Mar 20 at 15:49
$begingroup$
Like I said, geologists don't. Perhaps it has to do with the general 'noisiness' of geoscience datasets. Most other sciences are able to rely on fairly precise numbers.
$endgroup$
– geordie
Mar 20 at 16:22
1
$begingroup$
"Most other sciences are able to rely on fairly precise numbers." Ha, ha. You're funny!
$endgroup$
– JimB
Mar 20 at 16:30
|
show 2 more comments
2
$begingroup$
My usual soapbox speech: Why not consult a statistician first and then figure out how to implement an appropriate process in Mathematica? Consider stats.stackexchange.com/questions/267014/….
$endgroup$
– JimB
Mar 20 at 14:53
$begingroup$
@JimB precisely because someone will tell me that I can't use continuum methods with compositional data. The constant sum problem is considered a vague oddity in geological circles and largely ignored (for better or worse).
$endgroup$
– geordie
Mar 20 at 15:26
$begingroup$
I'm sure there must be more to it than "They won't let me do what I want!" Compositional data analysis has a pretty standard set of statistical approaches. Chemists use it all the time. These approaches are many times called "mixture designs": itl.nist.gov/div898/handbook/pri/section5/pri54.htm. Maybe you've been talking to the wrong folks.
$endgroup$
– JimB
Mar 20 at 15:49
$begingroup$
Like I said, geologists don't. Perhaps it has to do with the general 'noisiness' of geoscience datasets. Most other sciences are able to rely on fairly precise numbers.
$endgroup$
– geordie
Mar 20 at 16:22
1
$begingroup$
"Most other sciences are able to rely on fairly precise numbers." Ha, ha. You're funny!
$endgroup$
– JimB
Mar 20 at 16:30
2
2
$begingroup$
My usual soapbox speech: Why not consult a statistician first and then figure out how to implement an appropriate process in Mathematica? Consider stats.stackexchange.com/questions/267014/….
$endgroup$
– JimB
Mar 20 at 14:53
$begingroup$
My usual soapbox speech: Why not consult a statistician first and then figure out how to implement an appropriate process in Mathematica? Consider stats.stackexchange.com/questions/267014/….
$endgroup$
– JimB
Mar 20 at 14:53
$begingroup$
@JimB precisely because someone will tell me that I can't use continuum methods with compositional data. The constant sum problem is considered a vague oddity in geological circles and largely ignored (for better or worse).
$endgroup$
– geordie
Mar 20 at 15:26
$begingroup$
@JimB precisely because someone will tell me that I can't use continuum methods with compositional data. The constant sum problem is considered a vague oddity in geological circles and largely ignored (for better or worse).
$endgroup$
– geordie
Mar 20 at 15:26
$begingroup$
I'm sure there must be more to it than "They won't let me do what I want!" Compositional data analysis has a pretty standard set of statistical approaches. Chemists use it all the time. These approaches are many times called "mixture designs": itl.nist.gov/div898/handbook/pri/section5/pri54.htm. Maybe you've been talking to the wrong folks.
$endgroup$
– JimB
Mar 20 at 15:49
$begingroup$
I'm sure there must be more to it than "They won't let me do what I want!" Compositional data analysis has a pretty standard set of statistical approaches. Chemists use it all the time. These approaches are many times called "mixture designs": itl.nist.gov/div898/handbook/pri/section5/pri54.htm. Maybe you've been talking to the wrong folks.
$endgroup$
– JimB
Mar 20 at 15:49
$begingroup$
Like I said, geologists don't. Perhaps it has to do with the general 'noisiness' of geoscience datasets. Most other sciences are able to rely on fairly precise numbers.
$endgroup$
– geordie
Mar 20 at 16:22
$begingroup$
Like I said, geologists don't. Perhaps it has to do with the general 'noisiness' of geoscience datasets. Most other sciences are able to rely on fairly precise numbers.
$endgroup$
– geordie
Mar 20 at 16:22
1
1
$begingroup$
"Most other sciences are able to rely on fairly precise numbers." Ha, ha. You're funny!
$endgroup$
– JimB
Mar 20 at 16:30
$begingroup$
"Most other sciences are able to rely on fairly precise numbers." Ha, ha. You're funny!
$endgroup$
– JimB
Mar 20 at 16:30
|
show 2 more comments
2 Answers
2
active
oldest
votes
$begingroup$
One approach is to reframe it as a minimization problem:
xVec = Array[x, 13];
NMinimize[Total[(a.xVec - b)^2], Total[xVec] == 1, Thread[xVec >= 0], xVec]
You can also get an improved residual by relaxing the equality constraint:
NMinimize[Total[(a.xVec - b)^2] + (Total[xVec] - 1)^2, Thread[xVec >= 0], xVec]
Thanks to Roman for some simplifications.
$endgroup$
$begingroup$
Thread[xVec > 0]
would be simpler on the third line and does the same thing. Also, you can constrain the sum by equality:NMinimize[(a.xVec-b).(a.xVec-b), Total[xVec]==1, Thread[xVec>0], xVec]
. I think the square inTotal[(a.xVec - b)]^2
should be taken inside of theTotal
, not outside.
$endgroup$
– Roman
Mar 20 at 14:15
add a comment |
$begingroup$
This can be done as a linear programming problem although the optimization is not least-squares in that case. The idea is to set up new variables, which are absolute values of discrepancies between a.x.
and b
. This can be done as below.
xvec = Array[x, Length[a[[1]]]];
dvec = Array[d, Length[a]];
lpolys = a.xvec - b;
ineqs = Flatten[Thread[xvec >= 0], Thread[dvec - lpolys >= 0],
Thread[dvec + lpolys >= 0], Total[xvec] == 1];
Now use NMinimize
.
min, vals =
NMinimize[Total[dvec], ineqs, Join[xvec, dvec], PrecisionGoal -> 10]
Total[xvec /. vals]
(* Out[90]= 0.627674050324, x[1] -> 0.339337833732,
x[2] -> 0.292918812222, x[3] -> 0.195908896153,
x[4] -> 0.10491683141, x[5] -> 0.0591148361052, x[6] -> 0.,
x[7] -> 0., x[8] -> 0.00181140763364, x[9] -> 0., x[10] -> 0.,
x[11] -> 0., x[12] -> 0., x[13] -> 0.00599138274489, d[1] -> 0.,
d[2] -> 0., d[3] -> 0.502611255236, d[4] -> 0.,
d[5] -> 0.0178984583702, d[6] -> 0.0717916527728, d[7] -> 0.,
d[8] -> 0., d[9] -> 0., d[10] -> 0.0353726839451
Out[91]= 1. *)
$endgroup$
add a comment |
Your Answer
StackExchange.ready(function()
var channelOptions =
tags: "".split(" "),
id: "387"
;
initTagRenderer("".split(" "), "".split(" "), channelOptions);
StackExchange.using("externalEditor", function()
// Have to fire editor after snippets, if snippets enabled
if (StackExchange.settings.snippets.snippetsEnabled)
StackExchange.using("snippets", function()
createEditor();
);
else
createEditor();
);
function createEditor()
StackExchange.prepareEditor(
heartbeatType: 'answer',
autoActivateHeartbeat: false,
convertImagesToLinks: false,
noModals: true,
showLowRepImageUploadWarning: true,
reputationToPostImages: null,
bindNavPrevention: true,
postfix: "",
imageUploader:
brandingHtml: "Powered by u003ca class="icon-imgur-white" href="https://imgur.com/"u003eu003c/au003e",
contentPolicyHtml: "User contributions licensed under u003ca href="https://creativecommons.org/licenses/by-sa/3.0/"u003ecc by-sa 3.0 with attribution requiredu003c/au003e u003ca href="https://stackoverflow.com/legal/content-policy"u003e(content policy)u003c/au003e",
allowUrls: true
,
onDemand: true,
discardSelector: ".discard-answer"
,immediatelyShowMarkdownHelp:true
);
);
Sign up or log in
StackExchange.ready(function ()
StackExchange.helpers.onClickDraftSave('#login-link');
);
Sign up using Google
Sign up using Facebook
Sign up using Email and Password
Post as a guest
Required, but never shown
StackExchange.ready(
function ()
StackExchange.openid.initPostLogin('.new-post-login', 'https%3a%2f%2fmathematica.stackexchange.com%2fquestions%2f193638%2fdetermining-multivariate-least-squares-with-constraint%23new-answer', 'question_page');
);
Post as a guest
Required, but never shown
2 Answers
2
active
oldest
votes
2 Answers
2
active
oldest
votes
active
oldest
votes
active
oldest
votes
$begingroup$
One approach is to reframe it as a minimization problem:
xVec = Array[x, 13];
NMinimize[Total[(a.xVec - b)^2], Total[xVec] == 1, Thread[xVec >= 0], xVec]
You can also get an improved residual by relaxing the equality constraint:
NMinimize[Total[(a.xVec - b)^2] + (Total[xVec] - 1)^2, Thread[xVec >= 0], xVec]
Thanks to Roman for some simplifications.
$endgroup$
$begingroup$
Thread[xVec > 0]
would be simpler on the third line and does the same thing. Also, you can constrain the sum by equality:NMinimize[(a.xVec-b).(a.xVec-b), Total[xVec]==1, Thread[xVec>0], xVec]
. I think the square inTotal[(a.xVec - b)]^2
should be taken inside of theTotal
, not outside.
$endgroup$
– Roman
Mar 20 at 14:15
add a comment |
$begingroup$
One approach is to reframe it as a minimization problem:
xVec = Array[x, 13];
NMinimize[Total[(a.xVec - b)^2], Total[xVec] == 1, Thread[xVec >= 0], xVec]
You can also get an improved residual by relaxing the equality constraint:
NMinimize[Total[(a.xVec - b)^2] + (Total[xVec] - 1)^2, Thread[xVec >= 0], xVec]
Thanks to Roman for some simplifications.
$endgroup$
$begingroup$
Thread[xVec > 0]
would be simpler on the third line and does the same thing. Also, you can constrain the sum by equality:NMinimize[(a.xVec-b).(a.xVec-b), Total[xVec]==1, Thread[xVec>0], xVec]
. I think the square inTotal[(a.xVec - b)]^2
should be taken inside of theTotal
, not outside.
$endgroup$
– Roman
Mar 20 at 14:15
add a comment |
$begingroup$
One approach is to reframe it as a minimization problem:
xVec = Array[x, 13];
NMinimize[Total[(a.xVec - b)^2], Total[xVec] == 1, Thread[xVec >= 0], xVec]
You can also get an improved residual by relaxing the equality constraint:
NMinimize[Total[(a.xVec - b)^2] + (Total[xVec] - 1)^2, Thread[xVec >= 0], xVec]
Thanks to Roman for some simplifications.
$endgroup$
One approach is to reframe it as a minimization problem:
xVec = Array[x, 13];
NMinimize[Total[(a.xVec - b)^2], Total[xVec] == 1, Thread[xVec >= 0], xVec]
You can also get an improved residual by relaxing the equality constraint:
NMinimize[Total[(a.xVec - b)^2] + (Total[xVec] - 1)^2, Thread[xVec >= 0], xVec]
Thanks to Roman for some simplifications.
edited Mar 20 at 15:32
answered Mar 20 at 13:43


bill sbill s
55.1k377159
55.1k377159
$begingroup$
Thread[xVec > 0]
would be simpler on the third line and does the same thing. Also, you can constrain the sum by equality:NMinimize[(a.xVec-b).(a.xVec-b), Total[xVec]==1, Thread[xVec>0], xVec]
. I think the square inTotal[(a.xVec - b)]^2
should be taken inside of theTotal
, not outside.
$endgroup$
– Roman
Mar 20 at 14:15
add a comment |
$begingroup$
Thread[xVec > 0]
would be simpler on the third line and does the same thing. Also, you can constrain the sum by equality:NMinimize[(a.xVec-b).(a.xVec-b), Total[xVec]==1, Thread[xVec>0], xVec]
. I think the square inTotal[(a.xVec - b)]^2
should be taken inside of theTotal
, not outside.
$endgroup$
– Roman
Mar 20 at 14:15
$begingroup$
Thread[xVec > 0]
would be simpler on the third line and does the same thing. Also, you can constrain the sum by equality: NMinimize[(a.xVec-b).(a.xVec-b), Total[xVec]==1, Thread[xVec>0], xVec]
. I think the square in Total[(a.xVec - b)]^2
should be taken inside of the Total
, not outside.$endgroup$
– Roman
Mar 20 at 14:15
$begingroup$
Thread[xVec > 0]
would be simpler on the third line and does the same thing. Also, you can constrain the sum by equality: NMinimize[(a.xVec-b).(a.xVec-b), Total[xVec]==1, Thread[xVec>0], xVec]
. I think the square in Total[(a.xVec - b)]^2
should be taken inside of the Total
, not outside.$endgroup$
– Roman
Mar 20 at 14:15
add a comment |
$begingroup$
This can be done as a linear programming problem although the optimization is not least-squares in that case. The idea is to set up new variables, which are absolute values of discrepancies between a.x.
and b
. This can be done as below.
xvec = Array[x, Length[a[[1]]]];
dvec = Array[d, Length[a]];
lpolys = a.xvec - b;
ineqs = Flatten[Thread[xvec >= 0], Thread[dvec - lpolys >= 0],
Thread[dvec + lpolys >= 0], Total[xvec] == 1];
Now use NMinimize
.
min, vals =
NMinimize[Total[dvec], ineqs, Join[xvec, dvec], PrecisionGoal -> 10]
Total[xvec /. vals]
(* Out[90]= 0.627674050324, x[1] -> 0.339337833732,
x[2] -> 0.292918812222, x[3] -> 0.195908896153,
x[4] -> 0.10491683141, x[5] -> 0.0591148361052, x[6] -> 0.,
x[7] -> 0., x[8] -> 0.00181140763364, x[9] -> 0., x[10] -> 0.,
x[11] -> 0., x[12] -> 0., x[13] -> 0.00599138274489, d[1] -> 0.,
d[2] -> 0., d[3] -> 0.502611255236, d[4] -> 0.,
d[5] -> 0.0178984583702, d[6] -> 0.0717916527728, d[7] -> 0.,
d[8] -> 0., d[9] -> 0., d[10] -> 0.0353726839451
Out[91]= 1. *)
$endgroup$
add a comment |
$begingroup$
This can be done as a linear programming problem although the optimization is not least-squares in that case. The idea is to set up new variables, which are absolute values of discrepancies between a.x.
and b
. This can be done as below.
xvec = Array[x, Length[a[[1]]]];
dvec = Array[d, Length[a]];
lpolys = a.xvec - b;
ineqs = Flatten[Thread[xvec >= 0], Thread[dvec - lpolys >= 0],
Thread[dvec + lpolys >= 0], Total[xvec] == 1];
Now use NMinimize
.
min, vals =
NMinimize[Total[dvec], ineqs, Join[xvec, dvec], PrecisionGoal -> 10]
Total[xvec /. vals]
(* Out[90]= 0.627674050324, x[1] -> 0.339337833732,
x[2] -> 0.292918812222, x[3] -> 0.195908896153,
x[4] -> 0.10491683141, x[5] -> 0.0591148361052, x[6] -> 0.,
x[7] -> 0., x[8] -> 0.00181140763364, x[9] -> 0., x[10] -> 0.,
x[11] -> 0., x[12] -> 0., x[13] -> 0.00599138274489, d[1] -> 0.,
d[2] -> 0., d[3] -> 0.502611255236, d[4] -> 0.,
d[5] -> 0.0178984583702, d[6] -> 0.0717916527728, d[7] -> 0.,
d[8] -> 0., d[9] -> 0., d[10] -> 0.0353726839451
Out[91]= 1. *)
$endgroup$
add a comment |
$begingroup$
This can be done as a linear programming problem although the optimization is not least-squares in that case. The idea is to set up new variables, which are absolute values of discrepancies between a.x.
and b
. This can be done as below.
xvec = Array[x, Length[a[[1]]]];
dvec = Array[d, Length[a]];
lpolys = a.xvec - b;
ineqs = Flatten[Thread[xvec >= 0], Thread[dvec - lpolys >= 0],
Thread[dvec + lpolys >= 0], Total[xvec] == 1];
Now use NMinimize
.
min, vals =
NMinimize[Total[dvec], ineqs, Join[xvec, dvec], PrecisionGoal -> 10]
Total[xvec /. vals]
(* Out[90]= 0.627674050324, x[1] -> 0.339337833732,
x[2] -> 0.292918812222, x[3] -> 0.195908896153,
x[4] -> 0.10491683141, x[5] -> 0.0591148361052, x[6] -> 0.,
x[7] -> 0., x[8] -> 0.00181140763364, x[9] -> 0., x[10] -> 0.,
x[11] -> 0., x[12] -> 0., x[13] -> 0.00599138274489, d[1] -> 0.,
d[2] -> 0., d[3] -> 0.502611255236, d[4] -> 0.,
d[5] -> 0.0178984583702, d[6] -> 0.0717916527728, d[7] -> 0.,
d[8] -> 0., d[9] -> 0., d[10] -> 0.0353726839451
Out[91]= 1. *)
$endgroup$
This can be done as a linear programming problem although the optimization is not least-squares in that case. The idea is to set up new variables, which are absolute values of discrepancies between a.x.
and b
. This can be done as below.
xvec = Array[x, Length[a[[1]]]];
dvec = Array[d, Length[a]];
lpolys = a.xvec - b;
ineqs = Flatten[Thread[xvec >= 0], Thread[dvec - lpolys >= 0],
Thread[dvec + lpolys >= 0], Total[xvec] == 1];
Now use NMinimize
.
min, vals =
NMinimize[Total[dvec], ineqs, Join[xvec, dvec], PrecisionGoal -> 10]
Total[xvec /. vals]
(* Out[90]= 0.627674050324, x[1] -> 0.339337833732,
x[2] -> 0.292918812222, x[3] -> 0.195908896153,
x[4] -> 0.10491683141, x[5] -> 0.0591148361052, x[6] -> 0.,
x[7] -> 0., x[8] -> 0.00181140763364, x[9] -> 0., x[10] -> 0.,
x[11] -> 0., x[12] -> 0., x[13] -> 0.00599138274489, d[1] -> 0.,
d[2] -> 0., d[3] -> 0.502611255236, d[4] -> 0.,
d[5] -> 0.0178984583702, d[6] -> 0.0717916527728, d[7] -> 0.,
d[8] -> 0., d[9] -> 0., d[10] -> 0.0353726839451
Out[91]= 1. *)
answered Mar 20 at 14:34
Daniel LichtblauDaniel Lichtblau
47.6k277165
47.6k277165
add a comment |
add a comment |
Thanks for contributing an answer to Mathematica Stack Exchange!
- Please be sure to answer the question. Provide details and share your research!
But avoid …
- Asking for help, clarification, or responding to other answers.
- Making statements based on opinion; back them up with references or personal experience.
Use MathJax to format equations. MathJax reference.
To learn more, see our tips on writing great answers.
Sign up or log in
StackExchange.ready(function ()
StackExchange.helpers.onClickDraftSave('#login-link');
);
Sign up using Google
Sign up using Facebook
Sign up using Email and Password
Post as a guest
Required, but never shown
StackExchange.ready(
function ()
StackExchange.openid.initPostLogin('.new-post-login', 'https%3a%2f%2fmathematica.stackexchange.com%2fquestions%2f193638%2fdetermining-multivariate-least-squares-with-constraint%23new-answer', 'question_page');
);
Post as a guest
Required, but never shown
Sign up or log in
StackExchange.ready(function ()
StackExchange.helpers.onClickDraftSave('#login-link');
);
Sign up using Google
Sign up using Facebook
Sign up using Email and Password
Post as a guest
Required, but never shown
Sign up or log in
StackExchange.ready(function ()
StackExchange.helpers.onClickDraftSave('#login-link');
);
Sign up using Google
Sign up using Facebook
Sign up using Email and Password
Post as a guest
Required, but never shown
Sign up or log in
StackExchange.ready(function ()
StackExchange.helpers.onClickDraftSave('#login-link');
);
Sign up using Google
Sign up using Facebook
Sign up using Email and Password
Sign up using Google
Sign up using Facebook
Sign up using Email and Password
Post as a guest
Required, but never shown
Required, but never shown
Required, but never shown
Required, but never shown
Required, but never shown
Required, but never shown
Required, but never shown
Required, but never shown
Required, but never shown
ZdKkwQ54E4cSBh,DTA3aVglq1pDG,sLHm,nb ZVLBX,5cuZZQ
2
$begingroup$
My usual soapbox speech: Why not consult a statistician first and then figure out how to implement an appropriate process in Mathematica? Consider stats.stackexchange.com/questions/267014/….
$endgroup$
– JimB
Mar 20 at 14:53
$begingroup$
@JimB precisely because someone will tell me that I can't use continuum methods with compositional data. The constant sum problem is considered a vague oddity in geological circles and largely ignored (for better or worse).
$endgroup$
– geordie
Mar 20 at 15:26
$begingroup$
I'm sure there must be more to it than "They won't let me do what I want!" Compositional data analysis has a pretty standard set of statistical approaches. Chemists use it all the time. These approaches are many times called "mixture designs": itl.nist.gov/div898/handbook/pri/section5/pri54.htm. Maybe you've been talking to the wrong folks.
$endgroup$
– JimB
Mar 20 at 15:49
$begingroup$
Like I said, geologists don't. Perhaps it has to do with the general 'noisiness' of geoscience datasets. Most other sciences are able to rely on fairly precise numbers.
$endgroup$
– geordie
Mar 20 at 16:22
1
$begingroup$
"Most other sciences are able to rely on fairly precise numbers." Ha, ha. You're funny!
$endgroup$
– JimB
Mar 20 at 16:30